Overcoming Algocracy: AI, Blockchain and Crowdsourcing for Designing Better Dispute Systems
Join Federico Ast as he explores the promise, as well as the threat of algocracy and how crowdsourced solutions can be used to design better dispute systems.
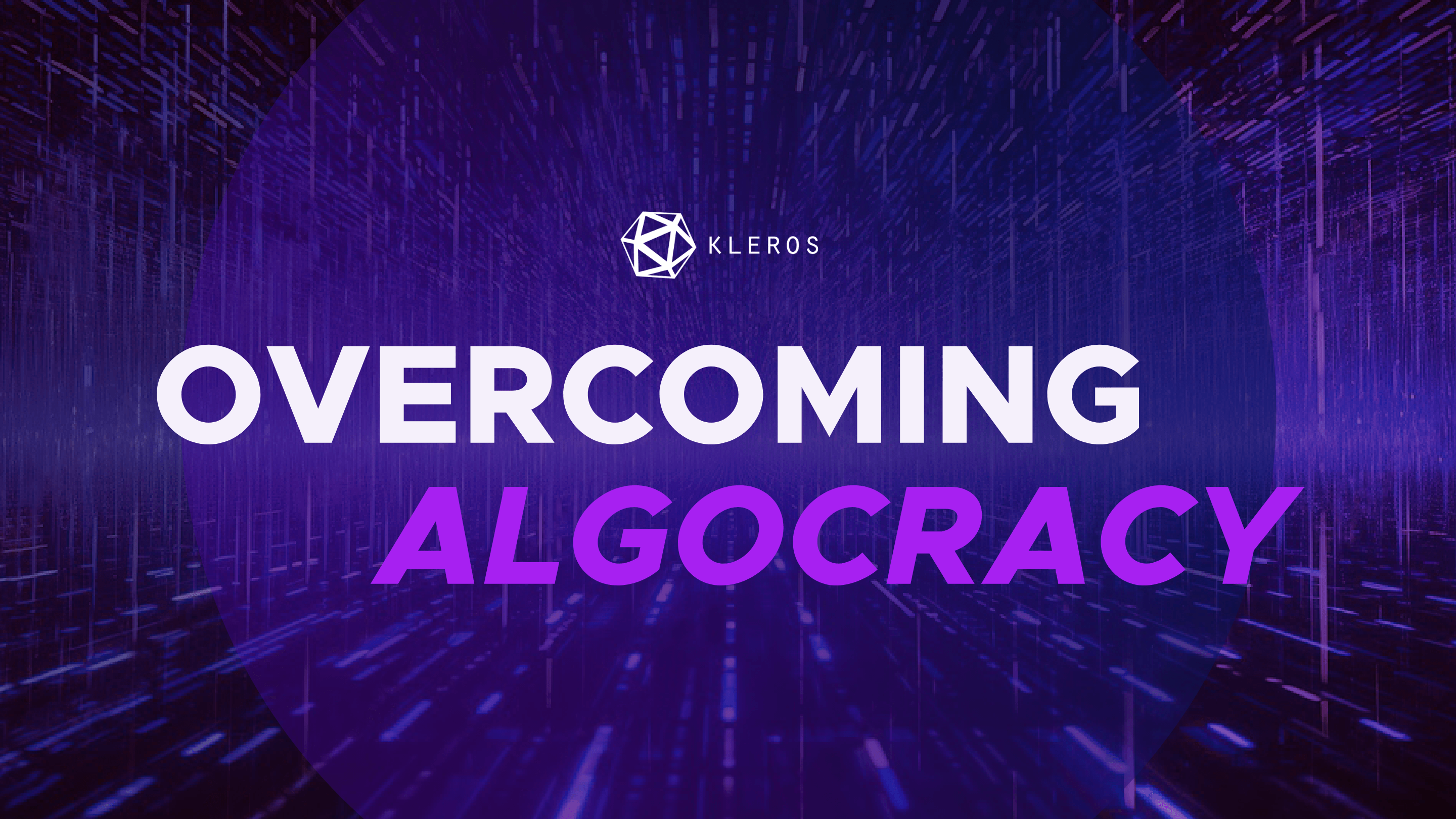
Previously published in the ABA Dispute Resolution November 2023 Just Resolutions Newsletter.
In May 2017, the police department of the British city of Durham announced the launch of the Harm Assessment Risk Tool (HART), an AI system designed to assist police officers in deciding whether a suspect should be released or detained in custody.
Trained on over 100,000 custody events, the algorithm was used to classify people arrested on suspicion of an offense as high, medium, or low risk of committing a crime in the future. Individuals assessed as low or medium risk were eligible for a rehabilitation program.
Those classified as high risk were kept in custody and had to face prosecution. Dr. Geoffrey Barnes, from the University of Cambridge, conducted a study on individuals arrested in Durham between September 2016 and October 2017. He followed the suspects to see whether they reoffended and returned to custody within two years.
HART proved to be slightly better than human officials at predicting reoffending rates. The HART model was accurate 53.8% of the time compared to a 52.2% accuracy rate for custody officers.
AI in Law: The Promise and The Peril
In the long run, any legal work that depends on analyzing historical data to predict likely outcomes will fall within the dominion of AI. Models similar to HART will be used to predict judicial decisions, arbitration rulings, and more.
Such systems can bring great benefits. They can help save time and cost for all parties involved, as well as provide higher accuracy data-driven results. But predictive machine learning models also face several challenges.
The first one is opacity. AI models are capable of making decisions, but not of explaining how they made them. HART proved to slightly outperform human officials in predicting the risk of reoffending.
However, it could not explain why any specific individual would be classified as
high or low risk. Decisions are made in a sort of "black box," and no reasons are given.
The second challenge is the risk of bias stemming from the dataset used to train the model. HART used 34 variables to predict the likelihood of reoffending, including age, gender, ethnicity, and place of residence.
The watchdog organization Fair Trials claimed that the model was biased against residents from poor neighborhoods, who were given a higher score of
reoffending risk.
The combination of opacity and bias could lead to a dystopian future of systems that makes potentially biased decisions without being able to explain them. This might be acceptable for an algorithm that makes personalized recommendations for TV shows on Netflix, where the worst that can happen is that the user won't like the recommended movie.
However, it can be problematic in the field of law.
One of the key elements of the rule of law is that justice doesn’t only need to be served; it also must be seen to be served by all parties involved and by people who are not legal experts.
Nobody Ever Got in Trouble for Following the Algorithm
Emerging regulatory frameworks for artificial intelligence, such as the AI Act of the European Union and the AI Bill of Rights of the United States call for strict human oversight of machine learning models.
Any AI decision tool should have an escalation mechanism where an appeal can be made to a human decision-maker. To be fair, HART was always intended to be a tool for decision support for human officials, not a robot judge that would make fully automated rulings.
However, as predictive systems become more widespread and accurate, a natural tendency could arise for human escalation mechanisms to start breaking down.
Imagine you are a custody officer having to decide the fate of a suspect. The algorithm has determined that this person has a high likelihood of reoffending, but you are unconvinced. After a hearing with the suspect, you honestly believe that she has decided to change and will not reoffend, so you decide to release her.
Two months later, this person commits murder. Your superiors (and potentially reporters) will want to know why you didn't follow the advice from the AI.
As they say in the business world, "Nobody ever got fired for buying from IBM." In the coming world of AI law, we could end up with a new saying: "Nobody ever got in trouble for following the algorithm."
Over time, the "safe decision" for human decision-makers will probably just be to go with whatever the AI has decided. Even if the algorithmic tools were just intended as support for decision-making, we might end up in a situation where they become, in practice, the main decision-makers.
Designing New Dispute Systems
In a debate organized by The Economist between author Yuval Noah Harari and
DeepMind founder Mustafa Suleyman, Harari claimed that the governance of AI will require the development of new institutions. Such institutions would need to provide human oversight in a way that ensures they are not themselves biased and do not end up surrendering all decision-making power to algorithms.
Even though we are still in the early stages, a human oversight system could be based on the principles of decentralized justice, a system that employs crowdsourcing and blockchain technology to render neutral and equitable decisions in disputes.
Such systems, of which Kleros is the first working example, assemble panels of expert jurors randomly to reach impartial decisions. The use of blockchain provides traceability, accountability, and transparency to the process. For example, by making sure that nobody can manipulate the data or the random draw
that determines which expert jurors should review a case.
An approach based on decentralized justice could address some issues in the context of human oversight of AI systems.
First, decentralized justice systems could establish an escalation mechanism where the outputs of AI models are continuously monitored for potential biases by a pool of jurors from diverse backgrounds. Jurors could be summoned to review the AI's decision-making process to determine if bias exists and to reverse decisions deemed unfair.
Second, the public nature of decentralized justice procedures adds transparency and accountability, which is especially important in a context where AI systems are opaque and cannot provide reasons for their decisions. Human jurors in decentralized justice processes can offer human interpretation. In cases where the juror panel agrees with the algorithm's decision, they can provide the necessary explanation to make the decision understandable to all parties.
Finally, decentralized justice systems could help audit AI models for biases. Models and datasets could be evaluated by a diverse group of jurors who would assess the system's fairness and flag any discriminatory patterns.
A decision-making system designed like this may help address the challenges of opacity and bias affecting AI models. The use of blockchain is crucial for all parties to verify that due process was followed, while crowdsourcing ensures a higher diversity of perspectives.
A larger number of jurors (maybe combined with the possibility of preserving their identity) could reduce the "just follow the algorithm" bias affecting individual decision-makers. Decisions made by this panel would be a source of feedback that would help AI developers in identifying and rectifying biases that may have been overlooked during development.
Overcoming the Threat of Algocracy
In the 19th century, philosopher Jeremy Bentham argued that the legal system was a mystery to the layman and needed more publicity and clear decision-making procedures.
Back then, the source of opacity came from the use of legal jargon and intricate procedures. In the future, it might come from the complexity of algorithms.
In their Wall Street Journal article "ChatGPT Heralds an Intellectual Revolution", Henry Kissinger, Eric Schmidt, and Daniel Huttenlocher argue that there is a fundamental problem for democratic decision-making if we rely on a system that is supposedly superior to humans but cannot explain its decisions. John Danaher called this "the threat of algocracy."
The forthcoming age of AI makes this issue even more relevant, as we are certain to have more automated systems making decisions that affect various aspects of our lives. We should not fear AI, as it has the potential to greatly improve the efficiency of legal systems and help us move towards the dream of justice inclusion.
However, to fulfill this promise, we need to place machine learning algorithms within an appropriate mechanism design to ensure that efficiency gains are not done at the expense of our democracies or our individual rights.
In this vein, dispute system designs including the diversity inherent in crowdsourcing and the transparency of blockchains can help us leverage the advantages of AI while minimizing the risk of algocracy.